[Computer World] The domestic and foreign data industries are facing innovation in data storage and management. Companies that have managed data using Data Warehouse (DW) and Data Lake (DL) are paying attention to “Data Lake House,” which combines the advantages of data Warehouse and data Lake, as a way to solve the difficulties of maintenance and management.Low cost and advanced management capabilities enable data management innovation
Key pillars of data technology, data warehouses and lakes
It was no exaggeration to say that the late 1980s was the era of data warehouses. The data warehouse used to help business decisions is a structured data analysis system that integrates data from multiple databases (DBs) and has long been a key part of the business intelligence (BI) process. This is because, unlike DBs that are dependent on individual systems, specific data can be integrated and refined according to the purpose and need, and used according to the purpose.
Finding data scattered in many places and integrating it into one has put a lot of manpower, time, and money. In the traditional BI process, building a data warehouse alone took about 80% of the time and cost. However, because there was no more effective data management methodology than data warehouses at the time, many companies jumped into building data warehouses.
However, in the late 2000s, problems that could not be addressed by data warehouses began to emerge. The biggest problem was that the amount and type of data in companies were increasing exponentially. Not all corporate data had useful value. We are faced with an ambiguous situation where we cannot invest in data warehouses that cost a lot of money to build or leave data untouched. Dataware houses, in particular, had “silo” where data within the organization was shared only by certain departments and not accessible by other departments.
It is Data Lake that has emerged as a solution to this problem. Data rake refers to a common data store that stores and shares data collected in various environments in its original, unprocessed form, that is, raw data. It is called a data lake because it is like a lake where all data, such as structured, unstructured, and semi-structured data, flows.
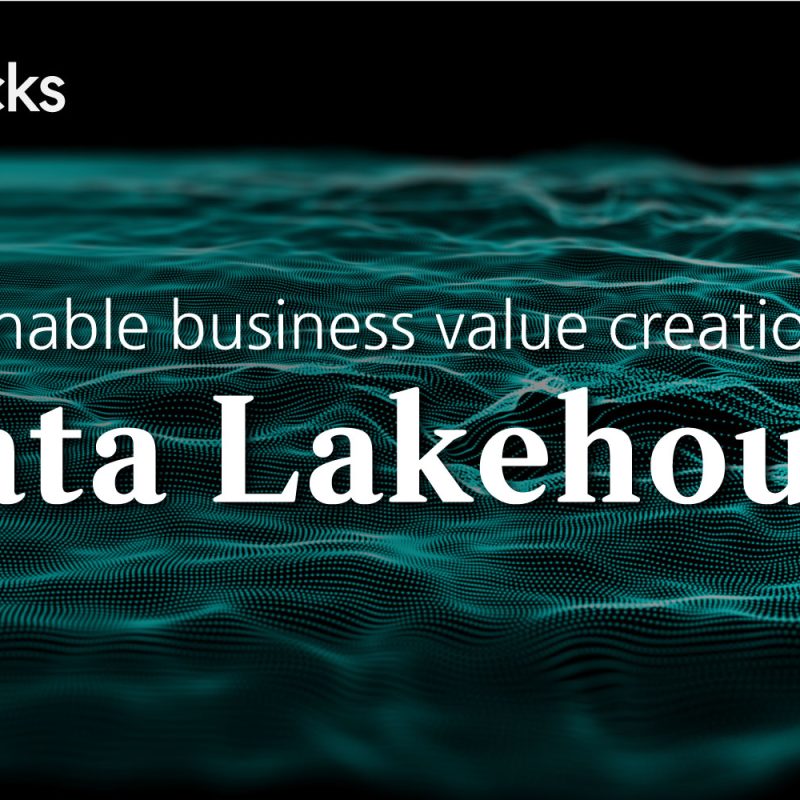
Data Storage Transformation (Source: Snowflake)
In particular, since there is no processing in the process of storing data, it was possible to store rapidly increasing data easily and quickly. In addition, it was very efficient to first collect the schema when storing data (Schemaless), and then define the schema on read when fetching the necessary data (Schema on read). In the case of data warehouses, specific schemas are defined when storing data.
Data warehouses require a separate dedicated system, but data lakes can be built with general-purpose hardware (HW) equipment, saving both time and money.
“Data Lake is a place to collect data, and data warehouses are a place to organize data neatly,” said consultant Kluckus Shim Jong-sung, summarizing the differences between the two technologies.
In fact, companies’ essential needs for data have not changed significantly over the past decade. It was to find useful insights while storing, integrating, and analyzing exponentially growing variety of data. This is why most companies operate two systems at the same time: multiple purpose-built data warehouses and a data lake that collects all data raw. However, it was difficult to fully satisfy users with data warehouses and data lakes.
Complex and difficult-to-manage dual data architecture
Both data warehouses and data lakes have distinct advantages. On the other hand, the shortcomings were also clear. Data lakes are advantageous for quickly collecting explosive data, but there was inevitably a problem in utilizing them as the source data was stored as it was.
In addition, data stored in data lakes require a lot of time and technology to process into raw data. In particular, if the data is not classified, it takes a lot of time to find the data you want.
If the user does not have enough capacity to handle the source data or takes too much time to process the source data for purpose, the data lake is inevitably a wrong choice. In this case, the data lake becomes a data dump or a data swamp where only data is collected.
In addition, data lakes are mainly intended to store data, and since raw data is stored as it is, there may be difficulties in terms of metadata management. Lack of information on the source, format, and frequency of updates of data may reduce data search and reliability, and access to data is also difficult.
Data warehouses have advantages in using data, but they take a lot of time to store data. In the case of data warehouses, data generated in real time cannot be utilized and are optimized for structured data, so they are not suitable for unstructured data processing required by the latest trend, machine learning.
It is also not cost-effective when it comes to storing large amounts of data. Even though it took a lot of time and money, data warehouses are difficult to use for other purposes than originally expected. It is difficult to obtain value beyond what was initially intended because only the data needed during the design process is selectively integrated. Whenever a new analysis request arises, a new data warehouse must be built and used accordingly. This is why as the number of data warehouses increases, data architecture becomes more complex and operational costs increase.
Using data warehouses and data lakes is also a huge burden for managers. If the data lake finds the desired data and replicates it to a data warehouse or the like, it costs a considerable amount of money and stores the same data in duplicate, resulting in a problem of complicating management points. In order not to make a once-built data warehouse obsolete, new data needs to be updated continuously, which also poses a cost and management burden.
Jang Jung-wook, head of the Korean branch of Databrix, said, “If it is distributed into two data platforms, Data Lake and Data Warehouse, various inefficiencies and problems will arise. First, there is no consistency in data management and inefficient duplication may occur. Data collected from multiple data sources are partially managed in the data lake and partially managed in the data warehouse, and sometimes overlapped with each other as needed for analysis. This not only increases the cost of redundant storage and conversion, but also leads to cases where it is difficult to know which version of data the derived KPI is based on,” he said.
He continued, “There are also problems in terms of data governance. Data rake generally manages data rights per file or folder. However, the data warehouse can manage access based on rows or columns. This means that when a user uses both systems, the precision of data access rights cannot be accurately managed. In addition to these problems, it is not easy to maintain a consistent data governance policy when using two different data management systems,” he said, pointing to the problems that arise when using both platforms at the same time.
In addition, Jang Jung-wook, the branch manager, said, “In addition, there may be difficulties in terms of collaboration.” Innovation through data analysis is essential to improve corporate competitiveness. However, collaboration between data scientists using data lakes and BI analysts who mainly use data warehouses is not easy, and it is difficult to expect innovative use cases. From the perspective of data engineers supporting these two groups, it is not easy to support and cooperate with different data systems, he added.
Data Lakehouse Combines Benefits Only
As mentioned above, data warehouses and data lakes have advantages but also limitations. It is the data lake house that comes as a way to overcome the limitations while maintaining the advantages of data warehouses and data lakes. The architecture of a data lake house is to integrate a layer that acts as a data warehouse over a data lake. Simply put, it is a way to solve shortcomings and save advantages. Data Lakehouse implements high-quality data management and structuring capabilities that data warehouses have, but they are realized on the flexible and affordable storage of data lakes, not on separate expensive data warehouse storage.
Data Lake House operates a new Lake House on the data Lake layer built on Amazon Web Services (AWS), Microsoft Azure, and Google Cloud. Since data is still stored in the data lake, advantages such as easy storage and low storage costs can be maintained. The source data in the data lake can be directly linked to BI tools even if the data is not duplicated to other stores, which also solves the problem that it is difficult to duplicate or update data, which was a disadvantage of the data warehouse.
Data Lakehouse has functions that can convert and manage unstructured data stored at the file level using “Apache Iceberg” or “Delta Lake” from the ACID (Atomic, Isolated, Consistent, Durable) transaction function and data management function that ensures data consistency and consistency.
In response, consultant Clukus Shim Jong-sung said, “It is possible to support major schemas such as Star schema and Snowflake schema. Even ACID transactions that were only applied to structured data or data genealogy management functions that were implemented as separate products can be applied to unstructured data, he said. “Data Lakehouse is built with an open-source architecture in data formats and APIs.”
Key technologies of Data Lakehouse include metadata layers, design of new query engines, and adoption of open data formats optimized for data science and machine learning tools. First, an open-source metadata layer such as Delta Lake is a technology that provides data management functions such as ACID transactions. It also has features such as streaming I/O support, regression to past table versions, forced schema application, and data validation.
Next, the technology that enables data rakehouses is the changed query engine design. In the past, access to data lakes was slow using cheap object storage. However, data rakehouses enable high-performance SQL analysis because they are vectorized and run on the latest CPU. Finally, it uses open data formats such as Apache Parquet. Open data formats such as Farkei are easy to access because they are compatible with Tensorflow and Pytorch, tools accessible to data scientists and machine learning engineers. In particular, Spark Dataframes can provide a declarative interface for open data formats to optimize additional I/O.
In response, Claudera Korea Executive Director Kim Ho-jung said, “Many big data projects today use Parkay, a column-based open data format, and since most distributed query engines or ETL tools support Parkay formats without being dependent on a specific language, data can be easily exported and shared. Data Lakehouse is built as an open-source-based architecture in data formats and APIs. Therefore, it is possible to effectively use various functions compared to data warehouses that are relatively friendly to a single company and consist of closed at first glance,” he added.
Data Lakehouse Enterprise Business and Solutions Strategy
Innovate data management with a data intelligence platform
Databricks is one of the companies that puts the most emphasis on Data Lakehouse. Databricks was founded by the founder of Apache Spark, which is indispensable in the open-source big data platform ecosystem, and has more than 7,000 customers around the world as a data lakehouse platform. It established a branch in Korea in April 2022.
Databricks is currently putting “Data Intelligence Platform” on the front of its business. Data intelligence platforms use AI models to innovate data management by deeply understanding the semantics of enterprise data. The data intelligence platform is built on Lakehouse, an integrated system that queries and manages all corporate data, but it can automatically analyze data (content and metadata) and how data is used (queries, reports, genealogies, etc.) to add new functions as needed.
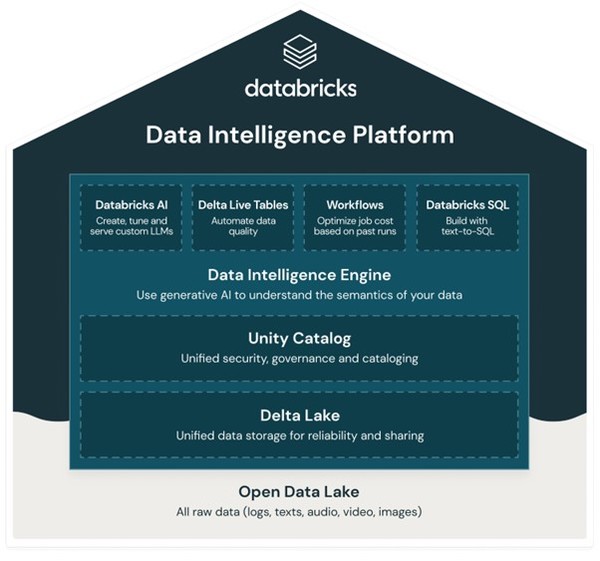
Databricks Data Intelligence Platform Architecture (Source: Databricks)
“Based on Lakehouse’s existing capabilities, Databricks has built a data platform with an integrated governance layer that encompasses data and AI, and a single integrated query engine that encompasses ETL, SQL, machine learning, and BI,” said Jang Jung-wook, head of Databricks Korean branch. We created an AI model in “DatabricksIQ,” a data intelligence engine that drives all parts of the platform through the recently acquired Mosaic ML (MosaicML),” adding, “The data platform was difficult for end users to access or for data teams to manage and control.” Databricks data intelligence platform expects to address these issues and transform the overall environment by enabling data to be queried, managed, and controlled much more easily. The fact that the data intelligence platform has a deep understanding of data and data use will be the basis for enterprise AI applications that operate based on data, he stressed.
“As AI reorganizes the SW industry today, only companies that strengthen their organizations by using data and AI in depth will be able to win the competition,” he said. The data intelligence platform will serve as a cornerstone for these organizations to develop next-generation data and AI applications with quality, speed and agility, he added.
[Cloocus Interview] “Creating Business Value with Data Lakehouse, Not Just Storage”
Q. What kind of business does Cloocus run in relation to data rakehouses.
A. Cloocus supplies Databricks’ Data Lakehouse platform, a solution within the Databricks platform that enables effective storage, management, and analysis of data.
This platform, with the distinctive features of Data Lakehouse, provides a comprehensive solution for integrated data management, large-scale data processing, ML/DL integration, data integrity, metadata management, real-time processing, and more.
H Company and E Company, Kluecous’ Databricks customers, effectively utilize the platform for various analysis tasks, starting from receipt data analysis to predictive services. Furthermore, it delivers excellent performance in data processing, seamlessly supporting ML Ops configuration by handling real-time data processing.
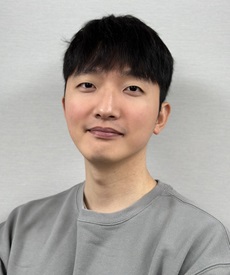
Cloocus consultant
Jong-sung Shim
Q. There are a number of data rakehouse products, and the direction of their development is.
A. There are currently various data rakehouse platforms. However, some solutions are in the process of evolving from a data warehouse platform to a data lakehouse, while others are evolving from a data lake platform to a data lakehouse. Each solution has its own advantages and disadvantages. Each solution will develop in the direction of strengthening its advantages and overcoming its disadvantages.
Personally, it is expected to make the process of using data rakehouses simpler and lower entry barriers to data analysis by incorporating AI technology. Only then will users who have difficulty writing code for data analysis be able to perform analysis effectively. I think future solutions should be developed to make data easier to use and to increase accessibility to data analysis through AI technology.
Q. Generative AI is in the spotlight, but is Data Lakehouse suitable for this too.
A. Regarding the functions for Generative AI, I think it can be helpful in terms of data processing. First, large-scale data processing capability is essential for Generative AI and data analysis. Real-time and high-performance data processing is important for fast data processing. You need data processing capability to work efficiently while processing and storing large data sets. The data lake house allows data to be effectively supplied to AI models and stored in storage.
Q. If you’re advising businesses and organizations looking to deploy data rakehouses..
A. By analyzing and leveraging data through a data lakehouse, organizations can achieve a variety of business goals, including discovering business insights, predicting customer behavior, and improving efficiency. The data is stored in the rakehouse, and if this data is not utilized, its value will not be fully derived. One of Lakehouse’s goals is to use data to gain insights and make strategic decisions.
Clarifying the purpose of using data is at the heart of data strategy. You need to define what questions you want to answer, what analyses you want to perform, what business goals you want to achieve, and collect, store, and process data accordingly. Only by designing and establishing this data-driven approach can data assets be utilized more efficiently and effectively.
Emphasize “Data Platform,” Expand Investment in Generative AI
Nowflake is also a representative player in the data lakehouse market. Data experts from Oracle gathered and co-founded, and gained fame in 2020 when Warren Buffett invested in public offering stocks in the process of listing on the New York Stock Exchange (NYSE). It established a branch in Korea in November 2021.
Snowflake started in a cloud data warehouse centered on SQL. As a data warehouse company, it means expanding to a data lake based on the data warehouse rather than adding a data lake to the existing data lake.
Although it is somewhat different from the data lake-based database method, it has the advantage of being able to access all data in the company and utilize the powerful functions of the data warehouse. Snowflake emphasizes the term Cloud Data Platform rather than the term Data Lakehouse.
Snowflake’s CDP solution has four major advantages: △ ease of use △ cost-effectiveness △ connected grid capabilities △ segmented governance. First is ease of use. Snowflake’s CDP is a fully managed service with automated management tasks such as platform construction, upgrades, storage maintenance, and execution engine provisioning. Users can only use it and leave the management to the snowflake side.
Next is high performance and cost-effectiveness. By using Python, SQL, Java, and Scala, structured, semi-structured and unstructured data can be processed into large data volumes, while simultaneously supporting multi-user requests without performance degradation. It continuously provides performance improvement and cost optimization through built-in performance optimization functions.
The third is that it has a grid function connected around the world. Any CSP whose execution environment is AWS, MS Azure, GCP, etc. provides one consistent user experience. Data can be securely connected in multi-cloud and cross-cloud environments to eliminate business silo phenomena, and new business models can be created.
The last is that you can specify granular governance. Data sensitivity, usage, and relationships can be understood as a whole and data can be protected through granular access control policies.
Specifically, a data classification scheme can detect and identify sensitive data and PII data, and object tags can be used to monitor sensitive data about compliance, retrieval, protection, and resource use. In addition, dynamic data masking policies can secure data, and tag-based masking policies can secure data.
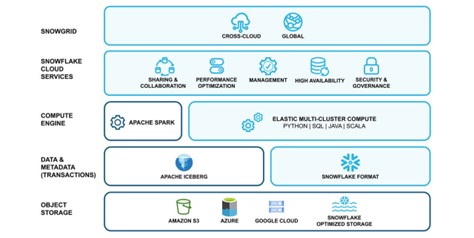
Snowflake’s Data Lakehouse Architecture (Source: Snowflake)
In addition, Snowflake also supports the function of supporting Generative AI. An official from Snowflake said, “We are helping companies break down data silos and apply more diverse functions. The field that Snowflake is currently concentrating and investing in is to easily and safely apply Generative AI to the corporate environment to provide new services. These include features that provide new value by providing various LLM models in Snowflake’s CDP environment, provide new user experiences based on built-in LLM, and increase user experience and productivity.
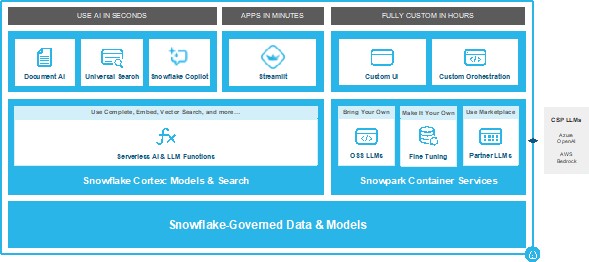
Snowflake’s Generative AI architecture (Source: Snowflake)
Open Data Lakehouse Offers ‘Cluedera Data Platform’
Claudera, an American company that provides services that support Apache Hadoop and Apache Spark-based SW, is also one of the companies attracting attention in the data lakehouse market. Claudera currently manages 25 exabytes of data for customers just like hyperscalers. Claudera’s data lakehouse core strategy is to provide an open data lakehouse, the Cloudera Data Platform, so that companies can use safe and reliable AI.
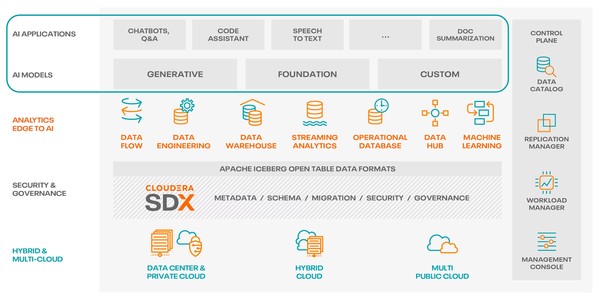
Structure of Claudera CDP (Source: Claudera)
“CDP, an open data lakehouse platform provided by Claudera, can apply any form of data to LLM through Apache Iceberg, a component, and helps more users across the organization use more data in more ways,” said Kim Ho-jung, executive director of Claudera Korea. “It is also the only hybrid data platform for the latest data architecture, unlike other public cloud-only suppliers.”
Iceberg is an open table format developed by Apache Software Foundation, which has the advantage that users are not dependent on suppliers. The official version can be used in CDP’s data services such as ‘Claudea Data Warehouse (CDW), ‘Claudea Data Engineering (CDE)’, and ‘Claudea Machine Learning (CML).
CDP is a hybrid data platform that provides open data rakehouses, providing multi-functional analysis of streaming and stored data through open cloud native storage formats across multiple clouds and on-premises. This allows the user to freely select a preferred analysis tool. In addition, integrated security and governance can be secured.
Companies with open data rakehouses can secure application interoperability and portability between on-premises and public clouds without worrying about data scaling. In addition, companies can leverage common metadata, security, and governance models in all data through shared data experience (SDX) built into CDP.
LG Uplus is a representative customer case. Claudera supported the establishment of a real-time big data analysis platform to secure the quality of 5G network services of LG U+. LG U+ has established ‘NRAP’, a network real-time analysis platform, through Claudera’s products and solutions to provide 5G network-based services to customers and increase work efficiency of network workers. This laid the groundwork for providing services to 20 million people in wired and wireless integration based on hundreds of types and hundreds of terra data generated by the communication network service group.
LG U+ NRAP refers to the entire system, including infrastructure that collects big data from all mobile terminals to service equipment related to telecommunication companies’ network services and provides them to be used as meaningful data. NRAP has increased analysis accuracy for data lakes and data warehouses, and it is highly utilized as it can process terabytes of data in up to seconds and send it to NMS, an integrated network management system. NRAP’s real-time data processing has improved customer quality response and service-based network quality monitoring to a level comparable to real-time, and achieved results such as improving customer satisfaction and reducing on-site work.
An overseas example is Japan’s Line. By using Apache Iceberg open data rakehouse and migrating workloads to CDP, Line was able to reduce operational issues by 70% while maintaining platform stability.
“Today, the digital economy is generating more and more types of data from more sources than ever before,” said Kim Ho-jung, executive director of Claudera Korea. As more and more companies deal with diverse data sources and hybrid environments, the need to implement data fabrics to safely operate different data sets and democratize data has increased, he said. “Claudea will help customers innovate data-driven digital by supporting the latest data technologies such as data mesh, data fabric, and data rakehouse so that companies can quickly move data from multiple environments and utilize it in real-time insights.”
See Related Articles : [Planning Special] ‘Data Lakehouse’ Combines DW and DL Advantages